COVID-19 Detection from Respiratory Sounds with Hierarchical Spectrogram Transformers
Jul 19, 2022·
,,,,,,·
0 min read
Idil Aytekin

Onat Dalmaz
Kaan Gonc
Haydar Ankishan
Emine U Saritas
Ulas Bagci
Haydar Celik
Tolga Cukur
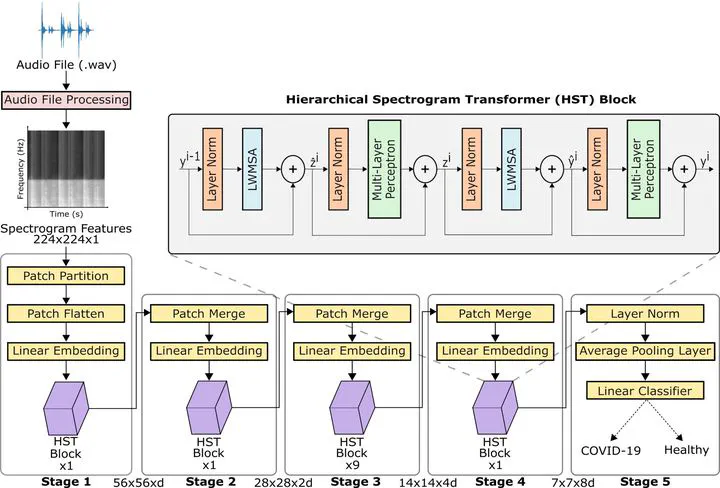
Abstract
Monitoring of prevalent airborne diseases such as COVID-19 characteristically involve respiratory assessments. While auscultation is a mainstream method for symptomatic monitoring, its diagnostic utility is hampered by the need for dedicated hospital visits. Continual remote monitoring based on recordings of respiratory sounds on portable devices is a promising alternative, which can assist in screening of COVID-19. In this study, we introduce a novel deep learning approach to distinguish patients with COVID-19 from healthy controls given audio recordings of cough or breathing sounds. The proposed approach leverages a novel hierarchical spectrogram transformer (HST) on spectrogram representations of respiratory sounds. HST embodies self-attention mechanisms over local windows in spectrograms, and window size is progressively grown over model stages to capture local to global context. HST is compared against state-of-the-art conventional and deep-learning baselines. Comprehensive demonstrations on a multi-national dataset indicate that HST outperforms competing methods, achieving over 97% area under the receiver operating characteristic curve (AUC) in detecting COVID-19 cases.
Type
Publication
under review, IEEE Transactions on Medical Imaging